There’s trillions of critters that live in the gut. But they don’t all just sit around each other, they form communities through the lining. Understanding the behavior of these communities and their formation is key to a more precise knowledge about the human gut microbiome. A team of biologists and engineers from the University of Michigan and University of Wisconsin have worked to create a new computer model that predicts the behavior patterns of millions of microbial communities.
“Whenever we increase the number of species, we get an exponential increase in the number of possible communities,” says co-corresponding author Alfred Hero, a professor of electrical engineering and computer science at Michigan, in a statement. “That’s why it’s so important that we can extrapolate from the data collected on a few hundred communities to predict the behaviors of the millions of communities we haven’t seen,” he adds.
Nowadays, more research continues to investigate how gut microbes influence human health. However, not much examines how consumption of probiotics and the subsequent introduction of a new bacterial strain impacts an existing microbe community. Machine learning and other artificial intelligence that can predict based on data sets may change this.
Ophelia Venturelli, assistant professor of biochemistry at Wisconsin and co-corresponding author of the study, along with her team, created hundreds of different microbial communities that are generally prevalent in the human gut. They monitored the evolution of them over time, carefully noting the concentrations of chemicals after food breaks down and relevant metabolites.
Through machine learning, the researchers were able to design communities with their desired metabolite compositions. By being able to control the environment this way, the technology could help doctors prevent and treat disease by introducing the correct microbes.
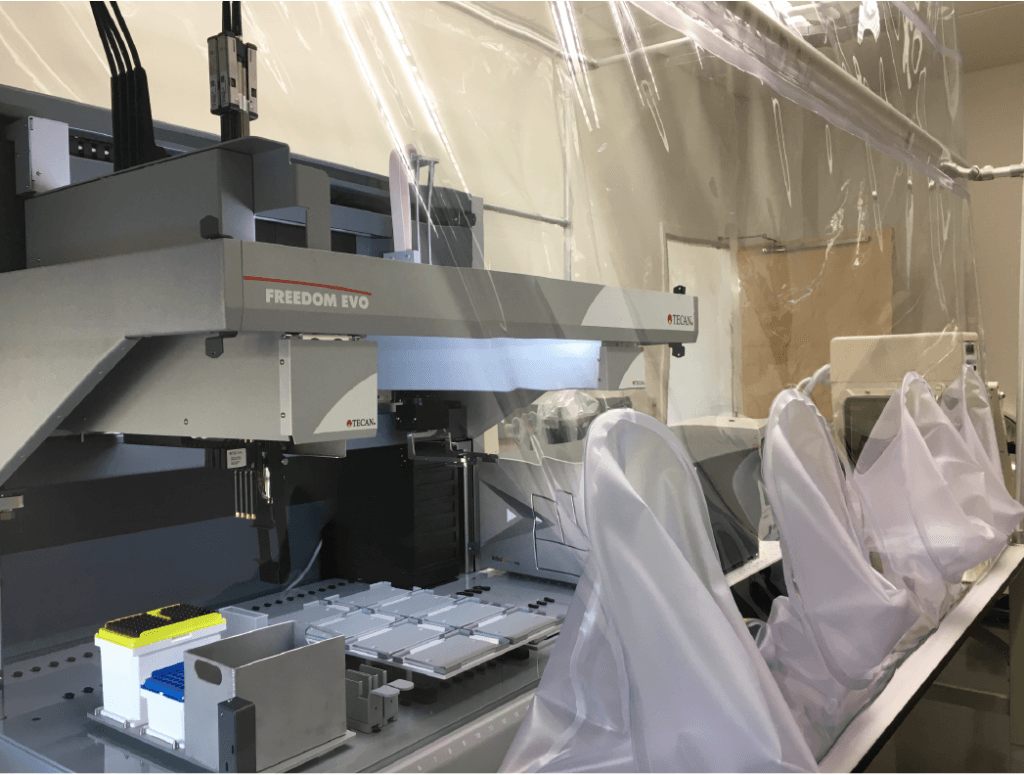
As for Hero’s team, they initially trained their algorithm using a data set from Venturelli’s lab. Similar to Venturelli’s, his team predict evolution and metabolite concentrations of newer communities constructed by her lab. Overall they had promising results, and their predictions were helpful for identifying where the model has room to improve, helping to enhance efficacy even more. “This new modeling approach, coupled with the speed at which we could test new communities in the Venturelli lab, could enable the design of useful microbial communities,” says Ryan Clark, co-first author of the study.
After analyzing results, the team agreed on a long short-term memory neural network for the machine learning algorithm, as it worked best for predictions about microbial behavior. By choosing an algorithm that best suits predictions, it strengthens the potential for doctors and other healthcare professionals to tailor gastrointestinal care in a more individualized way.
This study is published in the journal eLife.